Introduction
As technology is reshaping everything around us at the first pace, it is now more important than ever for businesses to make the most out of change.
Nothing is more powerful than data. It helps businesses analyze the past and present to better plan for the future.
Now, when we talk about data, data mining, and data science are the two things that come to mind.
It is quite popular terms that are often used interchangeably and are almost considered the same. But, that’s not the truth.
However, there are some similarities but a whole lot of differences as well.
In this blog, we will be considering the basics of data mining and data science, some common use cases, data mining vs data science differences, how these two work together to better help businesses, and one bust tip (that you don’t want to miss out on).
Let’s get into it.
What is Data Mining?
Data mining (also known as Knowledge Discovery in Data) is the procedure of collecting useful details, patterns, trends, hidden information, and relationships from raw data to make informed choices and projections. Data mining is merely one phase of data science, and it is regarded as a fundamental field in data science.
Data mining separates the data and assesses the likelihood of future events using complex mathematical algorithms. Data mining procedures employ a variety of services, including text mining, online mining, audio and video mining, graphical data mining, and social network data mining.
When executed efficiently, it improves corporate strategies and operations such as marketing, advertising, sales, customer service, manufacturing, supply chain management, human resources, finance, and much more.
- Customer Segmentation
Data mining helps businesses divide their customer base into distinct groups based on purchasing behavior, demographics, or preferences. This segmentation enables targeted marketing and personalized customer experiences. - Market Basket Analysis
It identifies relationships between products that are frequently purchased together. This insight is used for cross-selling, upselling, and designing product bundles to boost sales. - Fraud Detection
By analyzing transaction patterns and detecting anomalies, data mining helps identify and prevent fraudulent activities in industries like banking, insurance, and e-commerce. - Churn Prediction
Data mining identifies customers at risk of leaving by analyzing behavioral patterns and interactions. Businesses use these insights to create retention strategies and improve customer loyalty. - Sentiment Analysis
Data mining analyzes customer feedback, reviews, and social media posts to determine public sentiment toward a brand, product, or service. This helps businesses refine their offerings and communication strategies. - Predictive Maintenance
Most of the product-based businesses use varying types of equipment. And, data mining helps businesses in predictive equipment maintenance. It identifies patterns in sensor data to predict when equipment are likely to fail, reducing downtime and repair costs. - Risk Management
By analyzing historical data, data mining assesses potential risks in financial transactions, loans, or investments. It helps organizations mitigate risks and enhance decision-making. - Sales Forecasting
Businesses use data mining to analyze historical sales data, market trends, and seasonality to predict future sales. This enables better inventory management and strategic planning. - Supply Chain Optimization
Data mining identifies inefficiencies in logistics, inventory management, and supplier relationships. It helps businesses optimize their supply chains, reduce costs, and improve delivery times.
Also Read: How Data Analytics Helps Restaurant Grow
What is Data Science?
Data science is the study of enormous amounts of data; the data analyzed helps in the discovery of innovative solutions to challenging issues.
It is an interdisciplinary field that analyzes both organized and unstructured data to extract insightful information using a combination of tools, algorithms, and machine-learning concepts. To understand the complicated world of data, data scientists employ statistical, data analytics, and modeling approaches.
Data scientists are in charge of getting data, interpreting it, and connecting the resulting information and insights in such a way that they positively influence business choices.
Without data science, big data is meaningless. While big data drives hundreds of billions of dollars in spending across industries, bad data is predicted to cost the United States $3.1 trillion each year, highlighting the importance of data science. This loss can be converted into value by using data processing and analysis techniques.
Also Read: Business Intelligence and Data Analytics Services
What are the cases of Data Science?
- Predictive modeling: It is one of the most prevalent data science applications. It involves using statistical and machine learning algorithms to evaluate past data and find patterns and trends to create predictive models that can anticipate future events or actions.
- Fraud & Risk Detection: Data science not only helps in analyzing the data for fraud and risk detection, but it even detects fraudulent behavior and risk before it occurs, assisting firms with security breaches and protecting critical corporate data and resources.
- Business Intelligence: Data Science is frequently utilized in business intelligence to assist organizations in making data-driven choices. By studying massive datasets, companies can acquire significant insights into customer behavior, market trends, and other aspects that might help them formulate business strategies.
- Targeted Advertising: It assists companies in quickly identifying the ideal audience for advertising or promotion. Data scientists analyze digital marketing algorithms and verify purchases to ensure that the right individuals are seeing the goods, thereby helping collect consumer databases. It saves the marketer time and money by identifying a specific audience interested in the message they are delivering.
- Systems of Recommendations: Recommendation systems employ data science to make content, service, and product recommendations based on user behavior and interests. It assists companies in providing tailored insights for desired outcomes.
- Big data: Data science is capable of managing big data and other complicated and sizable datasets. It makes use of tools and technology that make it possible to process and analyze large amounts of data, producing insights that can be put to use even when faced with daunting data challenges.
- Visualization of data: Data science employs data visualization techniques to provide data in a visually appealing form, making it easier to convey findings and trends to technical and non-technical stakeholders alike.
- Solving problems: Data science is primarily about applying data-driven ways to solve difficult problems, whether they are related to company operations, healthcare results, or user experience.
- Natural Language Processing (NLP): It is a subfield of data science that is used in a variety of applications, including chatbots and virtual assistants, sentiment analysis, and text summarization.
Also Read: How Can Data Analytics Improve the Measurement of Employee Performance
Data Science vs Data Mining: The Key Differences!
Now, while you are aware of the key use cases of data science vs data mining, it’s time to dig in & find out how it differs and helps businesses in their way. Let’s get started:
Definition | Data Science is a field of study that combines programming, statistics, and domain knowledge to analyze and use data effectively. | Data Mining is a method used to analyze large datasets and extract valuable patterns and insights. |
Purpose | It focuses on collecting, processing, and analyzing data to create tools or strategies for businesses or research. | It focuses on finding specific useful information from data to help businesses make better decisions. |
Goal | The goal is to develop data-based solutions, such as predictive models, AI tools, or business strategies. | The goal is to extract meaningful patterns or trends from data for immediate use. |
Type of Data | Works with all types of data, including structured, unstructured, and semi-structured. | Primarily works with structured data, which is organized and formatted. |
Relation | Data Science is a superset of Data Mining, including tasks like cleaning data, creating models, and visualizing results. | Data Mining is a subset of Data Science, focusing specifically on finding patterns within data. |
Focus | Focuses on the science and techniques behind data, emphasizing understanding and creating solutions. | Focuses on specific processes and steps to extract valuable insights from data. |
Use-Case | Predicts future trends, solves problems and helps in planning strategies for business growth. | Finds patterns and relationships in past data to improve decision-making. |
Outcome | Provides actionable insights for automation, customer engagement, and improving processes. | Identifies key patterns and trends to refine existing strategies. |
Scope | Covers all stages of data handling—collecting, cleaning, analyzing, and building models. | Focuses specifically on extracting insights from structured datasets. |
Application | Helps with customer predictions, supply chain optimization, fraud prevention, and personalized marketing. | Used in fraud detection, customer segmentation, and analyzing sales patterns. |
Business Value | Helps businesses grow by offering predictive insights and better decision-making tools. | Improves business operations by revealing trends and opportunities in existing data. |
Time Orientation | Future-focused, providing predictions and recommendations for upcoming challenges. | Past-focused, analyzing data to learn from historical trends and patterns. |
Also Read: Business Intelligence Vs Machine Learning
How Data Science and Data Analytics Work Together to Help Businesses?
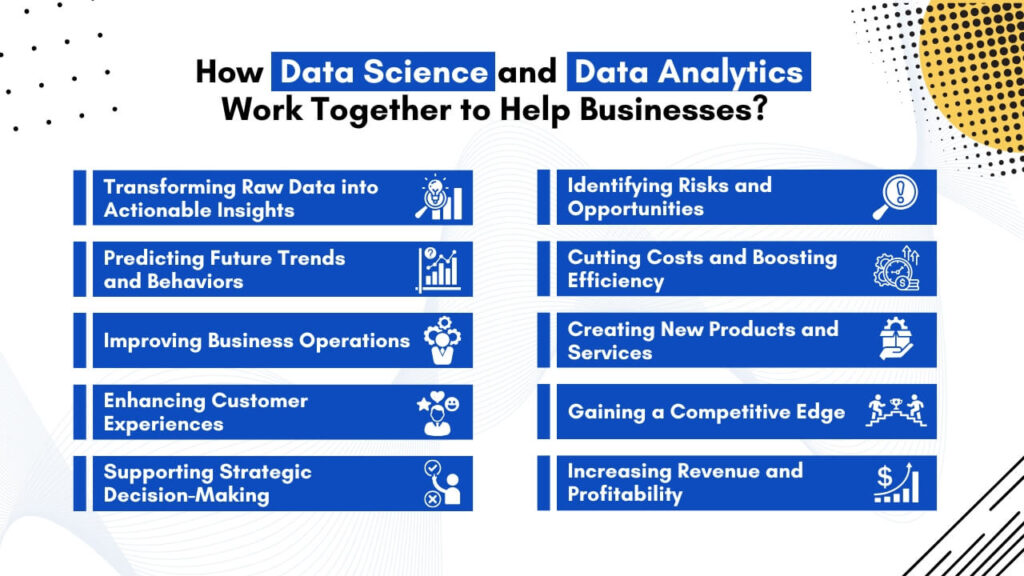
1. Transforming Raw Data into Actionable Insights
- Data Mining:
Data mining extracts valuable patterns and trends from large datasets by analyzing historical data. It identifies hidden insights such as customer preferences, product demand patterns, customer transactions, and other things that businesses can use to understand past behaviors and optimize strategies. - Data Science:
Data science takes the step ahead and leverages the insights found through data mining through advanced technologies like AI and machine learning to predict future trends and behaviors. It turns these insights into actionable strategies, helping businesses optimize operations, improve customer engagement, and forecast future needs.
Also Read: Benefits of Data-Driven Decision Making
2. Predicting Future Trends and Behaviors
- Data Mining:
Data mining identifies trends and patterns in historical data, such as identifying purchasing behaviors or customer segments. These insights give businesses an understanding of recurring events or trends, allowing them to better prepare for future changes.
- Data Science:
Data science then uses the patterns discovered by data mining to build predictive models. For instance, it predicts sales performance or customer responses to new products based on the data that has been accumulated over time. These predictive models help businesses plan, provide automated suggestions, and make data-driven decisions to meet future demands.
Also Read: How Predictive Intelligence Transforms Retail
3. Improving Business Operations
- Data Mining:
Data mining uncovers all the underlying operational data such as supply chain, customer service interactions, and other things that help businesses define the efficiencies, inefficiencies, and how the processes are working. All of these data and findings help businesses identify issues like delays, customer churn, negative customer feedback, higher wastage, and also what’s working enabling businesses to target areas for improvement. - Data Science:
Data science applies the insights from data mining to improve operations by creating tools like automation systems and AI-driven solutions. These automation, processes, and tools optimize operational processes like inventory management, customer management, supply-chain management, etc which helps in reducing costs, increasing efficiency, and improving customer experience as a whole.
4. Enhancing Customer Experiences
- Data Mining:
Customer experience is at the core of all the businesses. And, Data Mining comes to the rescue. It helps businesses collect and analyze customer data from various touch points like POS systems, websites, mobile apps, social media platforms, and social media. All of this collected data across various touch points helps businesses uncover patterns that are beneficial for businesses, enabling them to understand their needs and pain points better. - Data Science:
Data science takes these insights and personalizes the customer experience by using machine learning models to predict needs and recommend products. This helps businesses create more personalized customer experience, targeted marketing, and promotions which in turn helps in improving overall customer satisfaction, customer loyalty sales, and revenue.
Also Read: How AI Revolutionizes Customer Experience In Restaurant Industry
5. Supporting Strategic Decision-Making
- Data Mining:
Data mining uncovers hidden patterns, such as customer preferences or market trends, that help inform business strategies. It allows companies to understand their strengths, weaknesses, and opportunities. - Data Science:
Data science builds on these insights by visualizing data in easy-to-understand formats like dashboards and reports. It helps decision-makers clearly see the impact of different strategies and choose the best course of action based on data.
6. Identifying Risks and Opportunities
- Data Mining:
Data mining detects risks, such as fraud or market shifts, by analyzing patterns in historical data. It provides businesses with early warnings, allowing them to take action before issues escalate. - Data Science:
Data science uses predictive models to assess these risks and forecast future outcomes. It helps businesses identify new opportunities and risks, enabling proactive planning and informed decision-making to mitigate potential threats.
7. Cutting Costs and Boosting Efficiency
- Data Mining:
Businesses are always looking for ways to cut costs while still maintaining the quality of the products, services, customer experience, and top-notch satisfaction. Data mining helps businesses identify where businesses are spending most of their money, its total share of expenses in the businesses, how much the business is wasting, employee productivity, and other inefficiencies. - Data Science:
Data science takes and analyzes all the data and patterns that have been found through data mining and can be leveraged to take necessary actions to reduce costs without affecting the quality, experience, and process. Through data science, businesses can automate and even provide data-driven predictive intelligence to further help businesses save time, reduce operational costs, and improve overall business efficiency.
Also Read: How AI helps In Boosting Restaurant Revenue And Profits
8. Creating New Products and Services
- Data Mining:
Businesses are most of the time hesitant to launch new products and services wondering how it will benefit businesses and whether or not it will yield the required results. But, don’t worry. Data mining helps businesses identify gaps in the market by analyzing customer behavior, reviews, and purchasing patterns, and based on the previous customer experience and market trends – it even reveals unmet customer needs and businesses can provide what customers are looking for, guiding businesses in product development. - Data Science:
Data science uses insights from data mining to refine product ideas and predict their potential success. By testing concepts and optimizing features, businesses can create products and launch services that directly align with customer demand and market trends which helps businesses in getting the required returns.
9. Gaining a Competitive Edge
- Data Mining:
Another way in which data mining and data science work together to help businesses is by gaining a competitive edge. Data mining analyzes competitor data, such as market performance and customer feedback, to uncover trends and gaps in their strategies. This helps businesses understand their competition better and identify opportunities of what they can do better to differentiate and grab the right opportunities to win customers. - Data Science:
Data science takes competitor insights and uses predictive models to adjust business strategies accordingly. It helps companies make smarter decisions by comparing their performance with competitors, optimizing strategies, and staying ahead in the market.
10. Increasing Revenue and Profitability
- Data Mining:
Data Mining even helps businesses identify high-performing products, profitable customer segments, and effective marketing channels. These insights help businesses optimize their sales strategies and focus on areas that drive revenue growth. - Data Science:
Data science based on the data collected and with its predictive intelligence capabilities uses predictive models to forecast revenue growth and identify the best opportunities for maximizing profitability. It even combines market trends and customer behavior data for the best and accurate predictions, while driving revenue and profitability.
Bonus: Combine the Capabilities of Data Mining & Data Science with Livelytics!
We have listed the best use cases of data mining and data science and also happens when these two work together to help businesses. And, if you are looking to leverage the benefits of data mining and data science for your business – then Livelytics has got you covered.
Livelytics is a one-of-a-kind AI data tool with the capabilities of machine learning, business intelligence, big data, NLP, and predictive intelligence that helps businesses with data mining and data science.
It works as a data analyst and data scientist that helps you make the most out of your data and can help you set your business for success. So, what are you waiting for?
Book your free Livelytics demo & let’s get started.
To Conclude
Here’s the wrap on the comprehensive difference between data mining vs data science. We have added the basics of what is data mining and what is data science, how it differs, and how it works together to helps businesses regardless of the industry.
Also, you have checked that bonus tip as well, right?
So, if you would like to leverage the benefits of both data mining and data science, Livelytics is your go-to platform. It has the best features, best pricing and quite easy-to-use.
To know more about Livelytics and how it can further help you, book your free demo right away.
Frequently Asked Questions (FAQs)
We offer two pricing plans tailored to meet the diverse needs of businesses.
1. Our Standard Plan is priced at $299 per month, with an additional user fee of $10 per user per month. It includes essential marketing tools along with three AI-powered insights reports for inventory, customers, vendors, sales, and employees.
2. Our Premium Plan is available at $599 per month, with the same additional user fee. This plan provides access to advanced marketing tools and six AI-powered insights reports for inventory, customers, vendors, sales, and employees.
You know the best part? You can try either plan for free with our one-month trial offer.
As we stated, Data mining and data science have different capabilities. So, it is based on your business requirements. And, why choose one when you can have both? You can leverage the power of both data mining and data science with just one platform. If you would like to check it out, book a free Livelytics demo right away.
Yes, data mining is considered a subset of data science. Data mining is a critical step in the data science process, as it lays the foundation for further analysis and predictive modeling. Data mining deals with identifying patterns and trends in structured data, data science extends this by using those patterns to build predictive models and solve complex problems.
Livelytics enables seamless integration with your existing data sources, providing real-time, predictive insights. Also, it combines the capabilities of business intelligence, machine learning, NLP, and big data to predict the future better and set your business for success.
No, you need to hire a data analyst and data scientist after getting your hands on Livelytics. It is quite easy to get started with and use for you and your business. You can simply set it up, integrate and it’s done. You can do data ingestion, data analyses, and data transformation to better understand data and predict the future within just few clicks.