Introduction
Companies attempting to capitalize on the AI craze have hastily rebranded ML products as AI. Still, there is some fuzziness regarding the distinctions between these two technologies.
People in the technology industry frequently use the terms Artificial Intelligence and Machine Learning interchangeably. However, while AI and machine learning are closely connected, they differ in practically every way.
Are you trying to figure out what makes machine learning and artificial intelligence different from one another? Read on.The internet is filled with definitions that fail to correctly define AI and ML, which leads to confusion.
On the other hand, this site provides a thorough explanation of the differences between machine learning and artificial intelligence. So much so that even a non-technical individual will be able to differentiate between these concepts.
Furthermore, you’ll learn about the benefits of AI and machine learning in several business fields, which will help you gain a better understanding. So, read everything.
What is Artificial intelligence?
Artificial intelligence is a vast branch of computer science that focuses on developing intelligent computers capable of doing activities that would normally need human intelligence.
These responsibilities include making judgments and resolving difficult problems, as well as comprehending natural language and identifying patterns.
Artificial Intelligence has become a crucial component of company growth. In fact, companies using AI are predicted to see a 25% increase in customer satisfaction by 2023, and 91.5% of top companies regularly invest in AI.
Despite the fact that artificial intelligence is frequently conceptualized as a system unto itself, it is actually a collection of technologies incorporated into a system to allow it to reason, learn, and act in order to solve a complicated problem.
What is Machine Learning?
Machine learning is a subset of artificial intelligence.Contrary to artificial intelligence, machine learning does not refer to the development of intelligent robots or computer programs that can mimic human behavior to solve issues.
It includes building computer programs that, with the help of vast amounts of data and experiences, may learn and advance on their own without the need for human input.
For example, formerly, the assistants’ sole function was to identify particular statements, such as “What is the weather outside?” and provide an output. If anything more is said, the assistant is unable to generate the required results.
However, thanks to machine learning, assistants can now detect and respond to a wide range of statements.
Predictive analytics is another aspect of machine learning.
Several organizations, including Netflix and Amazon, employ predictive analytics to forecast customer behavior and provide a better user experience. Furthermore, banks employ predictive intelligence to identify fraudulent trends and prevent illegal activities.
To put it briefly, if artificial intelligence (AI) is the lofty goal of building intelligent computers or machines, machine learning (ML) is the set of models, tools, and procedures that professionals use to get there.
Getting Started with AI & ML
The Purpose
AI’s main goal is to make it possible for organizations to analyze and comprehend data in order to make better decisions. AI focuses on answering queries like “What happened?” And then “Why did it happen?”
ML, on the other hand, is primarily concerned with developing algorithms and models capable of making data-driven predictions or judgments. ML aims to provide answers to queries like “What should we do to achieve a specific outcome?” and “What is likely to happen in the future?”
And, when combined together these sophisticated and powerful technologies can do wonders for businesses when it comes to leveraging data, making decisions, and growing businesses.
Data requirements:
AI is commonly used for the analysis of structured data, including website traffic, customer profiles, and sales figures. AI tools are intended to operate with readily available, well-organized data.
In contrast, machine learning (ML) is often used to analyze unstructured data such as text, photos, and video.
To learn and produce accurate predictions, machine learning algorithms require a significant amount of data. Before this data can be utilized to train an ML system, it needs to be appropriately labeled and prepared.
Results
AI outputs are often visualizations, like as charts and graphs, which aid in the communication of data in an understandable format. AI results are intended to address particular queries and offer information that can help with decision-making.
ML’s outputs are often predictions or choices produced automatically based on the patterns and relationships found in data. ML outputs are frequently used to optimize corporate operations, enhance consumer experiences, and discover new opportunities.
Differences Between AI and ML for Businesses!
Aspect | Artificial Intelligence (AI) | Machine Learning (ML) |
Definition | AI refers to the simulation of human intelligence in machines that are programmed to think and learn like humans. It involves creating systems capable of performing tasks that require human intelligence. | ML is a subset of AI that focuses on developing algorithms and statistical models that enable machines to learn from and make predictions or decisions based on data. |
Scope | The scope of AI is comparatively broad and includes diverse fields such as robotics, natural language processing, computer vision, and cognitive computing. It aims to create intelligent systems that can perform a wide range of tasks. | Well, the scope of ML is narrower and it concentrates on data-driven approaches to enable machines to learn from data. It focuses on developing models that can perform specific tasks based on data patterns. |
Goal | To automate complex tasks, enhance decision-making, and simulate human-like intelligence in business processes. AI aims to achieve general intelligence and can be applied to a wide range of problems. | To enable systems to learn from historical data, identify patterns, and make informed predictions or decisions. ML aims to achieve specific intelligence and is applied to well-defined problems. |
Use Cases | Virtual customer service agents, personalized marketing, fraud detection, business process automation, predictive maintenance, and intelligent supply chain management. AI applications are often comprehensive and integrated into various business processes. | Customer segmentation, demand forecasting, recommendation engines, churn prediction, image and speech recognition, and predictive analytics. ML applications are often specific and targeted towards improving certain business functions. |
Techniques | AI often combines multiple techniques to achieve its goals.It includes rule-based systems, knowledge representation, expert systems, neural networks, genetic algorithms, and deep learning. | ML on the other hand, primarily employs supervised learning, unsupervised learning, reinforcement learning, and deep learning algorithms. ML focuses on finding patterns in data and making predictions based on those patterns. |
Impact on Business | AI can help businesses transform entire business operations by enabling new business models, revenue streams, and significant competitive advantages. AI can drive innovation and create new opportunities for businesses. | ML enhances specific business functions by improving efficiency, accuracy, and decision-making in targeted areas. ML can provide quick wins and measurable improvements in performance and productivity. |
Maintenance | It requires ongoing updates, monitoring, and adaptation to new data and scenarios to stay relevant and effective. | ML needs periodic retraining and validation to ensure model accuracy and relevance. It helps in incorporating new data and adapts to changing conditions. |
Data Requirements | It usually requires large and diverse datasets to train models effectively and ensure robust performance across various scenarios. Also, it often needs high-quality, annotated data to perform well. | ML also requires significant amounts of data, but the data can be more specific to the task at hand. ML models benefit from large, clean datasets but can start with smaller datasets and improve over time. |
Human Involvement | Often involves a higher level of human involvement in designing, training, and refining systems. AI projects may require interdisciplinary teams including data scientists, domain experts, and engineers. | Can involve less human intervention once the model is trained, although ongoing monitoring and maintenance are still necessary. ML projects can be managed by data science teams with domain knowledge. |
Adaptability | Highly adaptable to different tasks and can be applied across various industries and domains. AI systems can learn and adapt to new tasks with additional training and development. | More specialized, with models tailored to specific tasks and industries. ML models can be adapted to new tasks, but this often requires retraining and fine-tuning. |
Decision Making | Can provide more sophisticated and human-like decision-making capabilities, including reasoning, planning, and understanding complex contexts. AI systems can simulate cognitive functions and support strategic decision-making. | Focuses on data-driven decision-making, identifying patterns, and making predictions based on historical data. ML models excel in tactical decision-making and operational optimization. |
Also Read: How Do Machine Learning and Artificial Intelligence Helps Business
Best Use-Cases of AI & ML in Businesses!
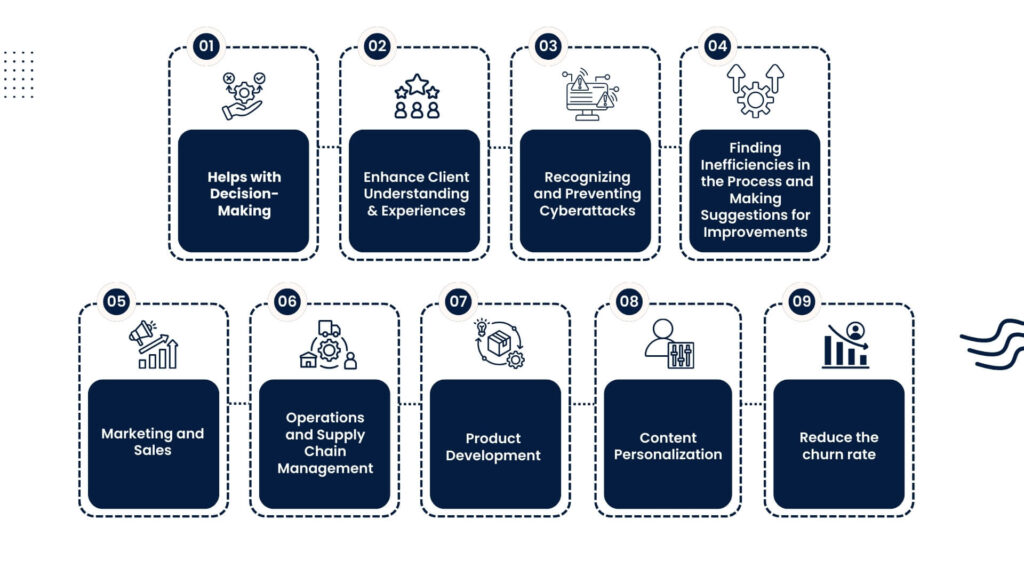
- Helps with Decision-Making
Business environments change quickly, so you risk falling behind if you wait for static reports. AI assists organizations in collecting all of their data, whereas ML uses predictive intelligence to assist enterprises in making data-driven decisions.
Advanced algorithms may evaluate data streams in real time, giving you with insights and recommendations. This enables you to respond swiftly to changing market conditions and client behavior, helping you stay ahead of the competition.
For example, a restaurant may utilize machine learning to offer appropriate items to clients in real time based on previous orders.
Also Read: AI to Collect Customer Insight In the Restaurant Industry
- Enhance Client Understanding & Experiences
AI may assist you in fully understanding your clients by evaluating historical purchase behavior, website behavior, and even social media sentiment.
With all the data, machine learning can uncover hidden client categories that would have been difficult to find otherwise. With this information, you may create more successful marketing and sales strategies and enhance the consumer experience.
“Organizations that can leverage their data to make informed decisions will emerge victorious in the future.” – Peter Sondergaard, a former Gartner Global Head of Research.
For instance, let’s say you own an online shoe business. ML can assist you in identifying a subset of consumers who value comfort over flashy looks. This informationWith marketing, you may use this information to target a certain demographic with your new line of ultra-padded sneakers.
- Recognizing and Preventing Cyberattacks
Our dependence on digital technologies has grown in the last several years. While it has undoubtedly made our lives easier, it has also increased our vulnerability to hacking and data breaches. Businesses become uneasy and lose their calm due to all of these assaults and beaches.
Business intelligence and machine learning, however, may be most helpful in identifying and thwarting cyberattacks before they start.
It can assist firms in recognizing and preventing emerging risks in the early detection phase, thereby saving critical consumer and business data.
- Finding Inefficiencies in the Process and Making Suggestions for Improvements
Many businesses are currently having trouble with static workflows, and the data that results from them is being underutilized.
Every organization has extremely distinct workflows. Machine learning can identify inefficiencies in processes as they are utilized and implemented.
Additionally, it might suggest process enhancements to boost organizational efficiencies across various teams as well as vertically and horizontally. This end-to-end process efficiency leads to better business operations and higher customer satisfaction.
- Customer Service and Support
AI-powered chatbots, enhanced with ML can seamlessly handle customer inquiries, providing instant responses and freeing up human agents for more complex issues.
These chatbots learn from each interaction, improving their accuracy and understanding of customer needs over time.
A case study on Bank of America’s AI-driven virtual assistant, Erica, shows how it handled over 100 million customer interactions in its first year, significantly improving customer satisfaction and reducing operational costs. Insane right?
- Marketing and Sales
Marketing has changed quite a lot. In these competitive times, customers are looking for targeted marketing efforts from businesses. And, AI & ML are at your rescue.
AI algorithms analyze vast amounts of customer data to create highly personalized marketing campaigns, while ML models predict customer behavior, enabling more targeted promotions and higher conversion rates.
Let’s understand how it works with an easy example:
Take an online retailer like Amazon. When a customer visits the site, AI looks at their browsing history and previous purchases.
Based on this data, ML predicts what the customer might want to buy next. If someone has been looking at running shoes and workout gear, the system might suggest new running shoes, fitness trackers, and workout clothes.
This information is used to show personalized recommendations on the website and in marketing emails. This targeted approach increases the chances that customers will buy the suggested items – leading to higher sales & revenue.
- Operations and Supply Chain Management
Managing the business operations & supply chain management is quite a hassle for businesses. It requires so much analyzing, and reporting, and it is time-consuming. Well, AI systems equipped with ML algorithms can help with that.
- AI monitors equipment, and ML predicts when maintenance is needed, reducing downtime.
- When combined, these technologies analyze logistics and forecast demand, improving stock management and cutting costs.
- AI tracks real-time inventory, and ML predicts future needs to prevent overstock and stock-outs.
- AI helps businesses optimize delivery routes, and ML predicts delivery times, improving customer satisfaction.
- AI evaluates supplier performance, and ML forecasts risks, ensuring a reliable supply chain.
- These technologies streamline production processes, improving efficiency and reducing waste.
- Product Development
AI helps businesses in product design by analyzing market trends, customer preferences, and feedback. ML models predict which features will be needed in the product, which helps businesses move in the right direction when it comes to product development.
For example, Nike uses AI to design new products based on customer feedback and market analysis, resulting in more popular product launches. “AI helps us understand our customers better and design products they truly want,” says Mike Parker, CEO of Nike.
When you have the right tools & technologies, all of it becomes easier.
- Content Personalization
AI analyzes user behavior to personalize content delivery, creating a more engaging and relevant experience for users.
ML algorithms can track and analyze what users watch, read, or listen to, and then suggest similar content that aligns with their preferences. This level of personalization keeps users engaged and encourages them to spend more time on the platform.
Spotify, for instance, uses AI to curate personalized playlists for its users. By analyzing the songs users listen to, skip, and save, Spotify’s algorithms can identify patterns in musical taste.
This information is used to create playlists like “Discover Weekly” and “Daily Mix,” which offer users a selection of songs tailored to their unique preferences.
According to Spotify, personalized playlists drive a significant portion of their listening hours, highlighting the effectiveness of AI in content personalization.
- Reduce the Churn Rate
ML models predict customer churn by analyzing purchase history, bookings, feedback, and engagement. These models can spot signs like fewer visits or negative reviews, indicating a customer might leave.
For instance, if a regular salon client skips appointments, the ML model flags them as at risk. The business can then offer personalized discounts, special promotions, or loyalty rewards to retain these customers. This proactive approach helps reduce churn and improve satisfaction.
To Conclude
We’ve explored how AI and ML can revolutionize your business, from understanding the basics to seeing how these technologies apply in real-world scenarios.
These tools can enhance customer experiences, streamline operations, and improve marketing efforts. But, to truly benefit from AI and ML, you need the right platform. That’s where Livelyticscomes in.
Livelytics is a powerful data platform that leverages AI and ML to give you clear, actionable insights tailored to your business, whether you’re in the restaurant, retail, hospitality, or salon industry.
Curious to see how it works? Try our free demo to experience how Livelytics can help you make smarter, data-driven decisions and take your business to the next level.
Book your demo today and start making the most of your data with Livelytics!
Frequently Asked Questions (FAQs)
In the competition between AI and machine learning, there is no clear winner or loser. After all, they are both useful, correct? It’s only that AI has a broader reach than Machine Learning, which concentrates on a specific task.
To ensure the seamless incorporation of AI and ML in your business processes, you need to begin by identifying key areas where AI and ML can add value. Also, you should consult with experts, choose the right tools and platforms, and start with a small pilot project to gradually integrate these technologies into your operations.
Livelytics is designed to integrate seamlessly with your existing systems, whether you use point-of-sale (POS) systems, booking software, accounting tools, or other operational tools to ensure a smooth transition and minimal disruption.
Data security and compliance are quite important for businesses. So, Livelytics implements robust security measures and complies with data protection regulations to safeguard your business data. We prioritize data privacy and ensure that your information is protected against unauthorized access.
Yes, Livelytics offers a free demo to potential customers. It helps you explore the platform’s features and capabilities and its compatibility with your business and industry before making a commitment to get the best out of your investment.